Staff
Andreas Scheidegger
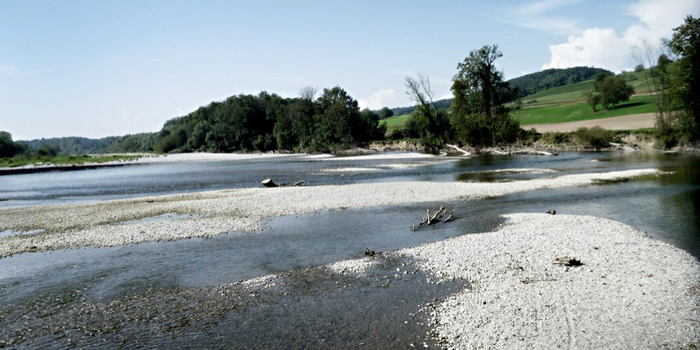
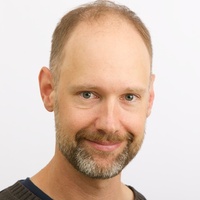
Andreas Scheidegger
Statistics, Data Science & Modeling
Department Systems Analysis, Integrated Assessment and Modelling
About Me
Research Interest
As a statistician, my focus is on using statistical techniques, machine learning, and applied mathematics to develop accurate models for examining scientific hypotheses and aiding decision making.
Mathematical modeling is a fundamental tool of science. Models are applied to extract knowledge from data, to combine available information, or to make predictions about the future states of a system. In any case models need to be tailored to the scientific questions at hand, so that all available information is utilized. This includes data but also system understanding and expert opinions.
In addition to the technical aspects of modeling, I also place a strong emphasis on effective communication, as it is crucial for successful collaboration. For successful application users must understand the underlying assumptions and limitations of a model.
Obtaining scientific data from field observations or experiments is a laborious and expensive task – my aim is to facilitate the best possible use of this hard-earned data.
Methods and Tools
Some topics and methods I work with or I am interested in:
- Bayesian Inference
- Gaussian Processes
- Data assimilation
- Machine Learning
- Artificial Neuronal Networks, Deep Learning
- Uncertainty Quantification
- Causal Inference
- Graphical (hierarchical) Models
For implementation I use among others Julia, R, Python, STAN, Emacs.
[[ element.title ]]
[[ element.title ]]
Curriculum Vitae
[[ entry.date || 'empty' ]] |
[[ element.title ]]
Publications
[[item.title]]
[[ element.title ]]
Files
[[ element.title ]]
Address
E-Mail: | andreas.scheidegger@cluttereawag.ch |
Phone: | +41 58 765 5053 |
Fax: | +41 58 765 5802 |
Address: | Eawag
Überlandstrasse 133 8600 Dübendorf |
Office: | FC D10 |
[[ element.title ]]
[[ element.title ]]
[[ element.title ]]
Research Group
[[ element.title ]]
Focalpoints
Statistical Modeling
Machine Learning and Data Science
Uncertainty Quantification
Bayesian Inference