Department Urban Water Management
FLOODvision
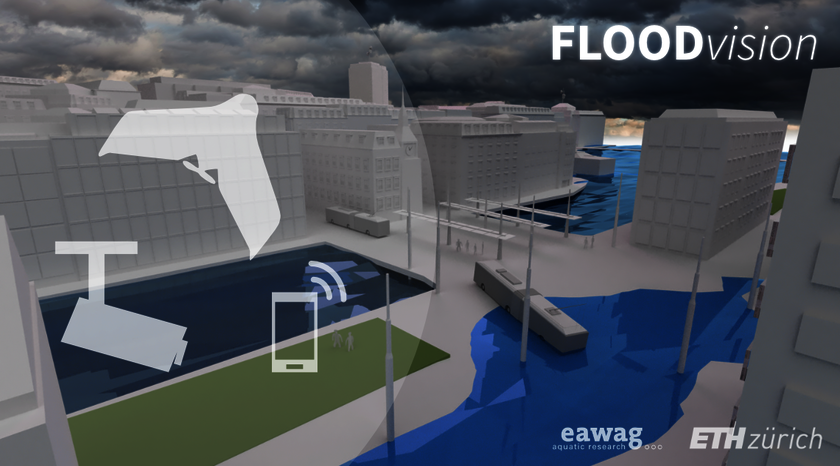
Image-based flood monitoring
Urban flood monitoring data are very hard or impossible to collect with conventional flow sensors. With recent advances in image analysis and deep learning, such data can be collected in near real-time from surveillance camera networks and social media.
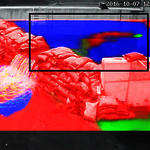
Probabilistic flood trend analysis from CCTV videos with convolutional neural networks
A deep convolutional neural network is trained on surveillance video frames to segment water-covered surfaces. Thanks to an index that qualifies the extent of flood coverage in each image, a data series can be computed from which the trends of the actual flood water level can be extracted. link to publication
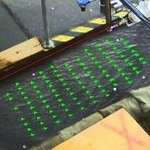
SSIV: Tracerless particle image velocimetry for urban runoff monitoring
Today, no sensors on the market are specifically designed for surface runoff in urban areas. In this project, consumer-grade surveillance cameras are used to determine velocity of shallow overland flow thanks to surface structure image velocimetry (SSIV), a type of Large Scale Image Velocimetry (LSPIV) optimized for tracerless flow thanks to special image filters and correlation analysis. This solution is a cost-effective use of existing infrastructure, and could revolutionize flood monitoring. link to publication
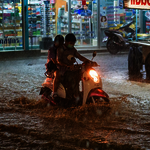
Semantics-based water level estimation from social media images
Social media images taken during floods often contain subjects whose approximate dimensions are known (e.g. cars, bicycles, or people). These objects, partially immersed in the flood water, serve as references for estimating water level. We developed an end-to-end classifier based on deep convolutional networks to automatically estimate water level. (Collaboration led by Dr. Jan Dirk Wegner) link to publication
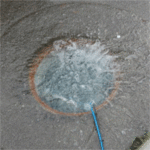
One of the main challenges in flood monitoring and modeling research is the availability of comprehensive datasets with which new methods can be developed and validated. The floodX flash flood experiments were conducted in a facility designed to train rescue personnel during urban flood facility. The result is a comprehensive urban flood database with multiple flood events and dense monitoring from diverse sensor systems. The data is open access. Read more...
Remote sensing layout data for urban drainage and flood models
Dual drainage models and coupled urban pluvial flood models require up-to-date and detailed layout data to correctly represent hydraulic and hydrologic phenomena. We explore new data collection methods that are scalable (street-level image services) and flexible (unmanned aerial vehicles).
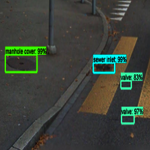
Automated asset mapping with street-level imagery
A convolutional neural network trained to detect manhole covers, sewer inlets, and valve covers in very high resolution Google Street View panoramas. The locations of the detected objects are then estimated thanks to panorama metadata and assumptions about data collection and the surrounding terrain. By exploiting already collected data, the approach is low-cost and easily expendable to regions where Google Street View is available. link to publication
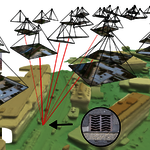
Multi-view asset mapping with UAV image clouds
A fully automatic framework for localizing small objects from image clouds captured from an unmanned aerial vehicle (UAV). Thanks to high image overlap of UAV images, a multi-view approach can be adopted to improve detection performance. The framework is demonstrated with a Viola-Jones classifier trained to detect sewer inlets at a ground sampling distance of 3.5 cm/pixel. Compared to single-view detection, multi-view detection improves both recall and precision. link to publication
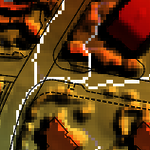
Detailed land use and elevation data collection with UAVs
Light-weight UAVs offer an easy-to-deploy and safe platform for collecting very high-resolution imagery of urban catchments. With machine learning, the imagery can be classified pixel-by-pixel into rough land use categories, for use in urban drainage models. Photogrammetric processing of the UAV images provides high-resolution elevation information for overland flow modelling. link to publication