Department Systems Analysis, Integrated Assessment and Modelling
Bridging the gap between data science and mechanistic modelling to gain knowledge about community assembly
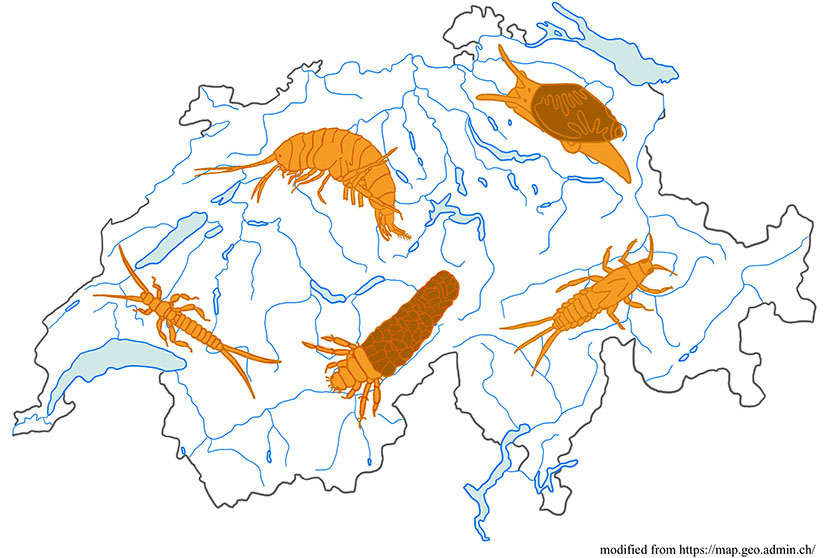
Background
Community assembly in ecosystems depends on various factors: On which species are present in the region, on local environmental conditions, and on the interactions between species. Different methods can be used to predict the occurrence probability of different species. These are either based on the mathematical formulation of existing knowledge about the mechanisms that influence species occurrence. Or they are based on statistical data analysis. In addition, there are machine learning methods, where it is more difficult to understand relations with environmental factors. All three methods have advantages and disadvantages, but have rarely been used together because they differ in their requirements for data and computational effort.
Content and aim of the research project
In this project, mechanistic models, statistical models, and machine learning methods will be applied to improve knowledge of environmental effects on macroinvertebrates in streams, so that in the future we can better predict which species occur under which environmental conditions. We will use the knowledge gained to develop a model that is as simple and efficient as possible, contains the most important mechanisms and is optimized for prediction. Another goal is to use the computer simulations to identify macroinvertebrate community metrics that are as sensitive as possible to future environmental changes.
Scientific and societal context of the research project
Our work will generate new insights for predicting community assembly that can inform the management of aquatic ecosystems in response to future environmental changes.