Department Environmental Chemistry
EXPECTmine
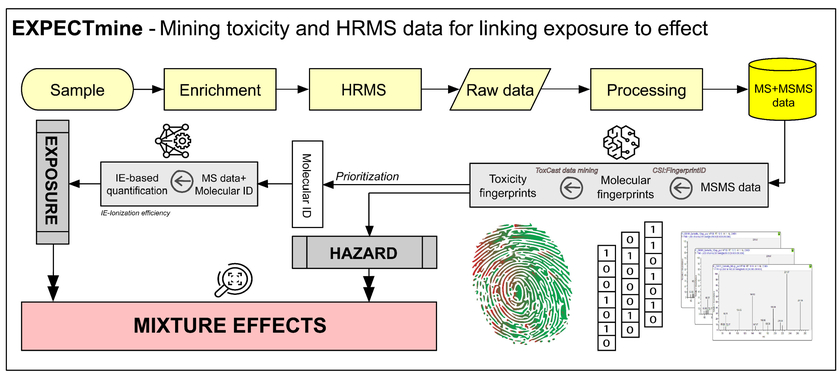
Identification and quantification of species in the vast and diverse universe of organic micropollutants is a daunting challenge for environmental sciences. Nontarget screening (NTS) based on high-resolution mass spectrometry (HRMS) has enabled the characterization of unknown compounds in complex matrices. However, a simple water sample typically contains thousands, or even tens of thousands, of signals, whose full elucidation is not feasible even with the most sophisticated data analysis workflows. In a typical approach, a subset of signals is selected for processing and identification based on various criteria, e.g., the particular research target or signals' abundance. Such practices are often biased toward a specific chemical space based on the prior knowledge applied during prioritization. In the EXPECTmine project, we utilize a battery of data mining techniques to develop a hazard-driven prioritization that combines species' exposure and potential effect. The model builds on an existing framework of machine learning tools for, e.g., standard-free quantification of species based on their ionization efficiency (Liigand et al. Scientific reports 10.1 (2020): 1-10.) and CSI:FingerID for predicting molecular fingerprints from MSMS data (Dührkop et al. PNAS 112.41 (2015): 12580-12585.). While MSMS and in-silico fragmenters are routinely applied in non-targeted workflows for structural elucidation, in EXPECTmine, they will be coupled to a data mining algorithm trained on high-throughput screening data from ToxCast (Richard et al. Chem Res Tox 29.8 (2016): 1225-1251.) and predicting species potential for toxicity. The developed tools will optimize and streamline the focus of non-targeted workflows towards species with a high potential to harm, as well as open up new opportunities for, e.g., assessing complex samples based on their overall hazard (effect and exposure). The developed workflow will be applied to complex water matrices such as wastewater.