Department Systems Analysis, Integrated Assessment and Modelling
Machine Learning & Complex Systems
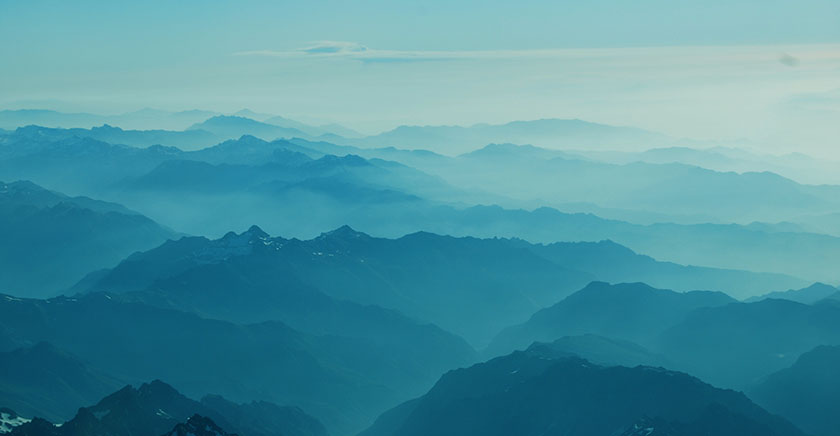
We use machine learning and statistical physics methods to tackle problems with large available datasets, and which involve a large number of interacting agents.
Ongoing research projects include:
- Exploring the impact of toxines on aquatic organisms, predicting the effect of untested toxines on the species, guiding toxicological assessments.
- The creation of a giant labeled dataset of plankton images and using it to infer the interactions among organisms, and between organisms and environment.
- The study of the long-time dynamics of high-dimensional systems, from toy models, to deep neural networks and ecosystems.