Department Systems Analysis, Integrated Assessment and Modelling
Parameter inference with stochastic models
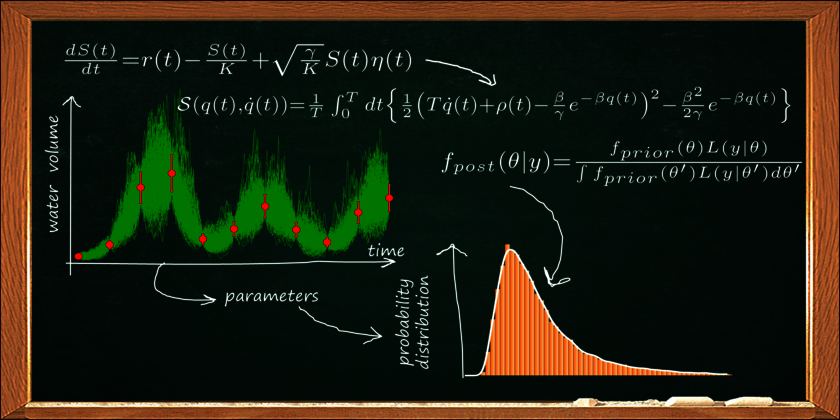
In order to understand, predict and possibly control the dynamics of complex systems, scientists design conceptual mathematical models that include the dominant system processes, state variables and parameters. Such dynamical models are described by differential equations. An essential prerequisite of a model to make reliable predictions on the behavior of a complex system is to appropriately account for all relevant sources of uncertainty. Thus, including the dominant errors in the model naturally leads to stochastic differential equation (SDE) models. A reliable quantification of parameter uncertainties is of fundamental importance to faithfully predict systems dynamics and bears a great potential which is still largely unexplored in many areas of environmental sciences.
Bayesian statistics describes our knowledge about parameters through probability distributions, interpreting them as the outcome of a data-driven learning process. This approach is especially well-suited for our purposes. We borrow well-known concepts and techniques from physics, such as path-integrals, molecular dynamics and Hamiltonian Monte Carlo and apply them to a Bayesian framework to develop novel methods to estimate model parameters and quantify their uncertainties on the basis of noisy time series of measurement data. The process is known as parameter inference. Our methods exhibit a much greater efficiency compared to classical Monte Carlo inference approaches. Moreover, high performance computing (HPC) methods such as automated differentiation and parallel computing substantially contribute to make our algorithms especially fast.
Although we initially focus on possible applications in hydrology, our methods are general and expected to be transferable to other fields, such as ecology, systems biology and engineering.